The Importance of Training Data for Self-Driving Cars
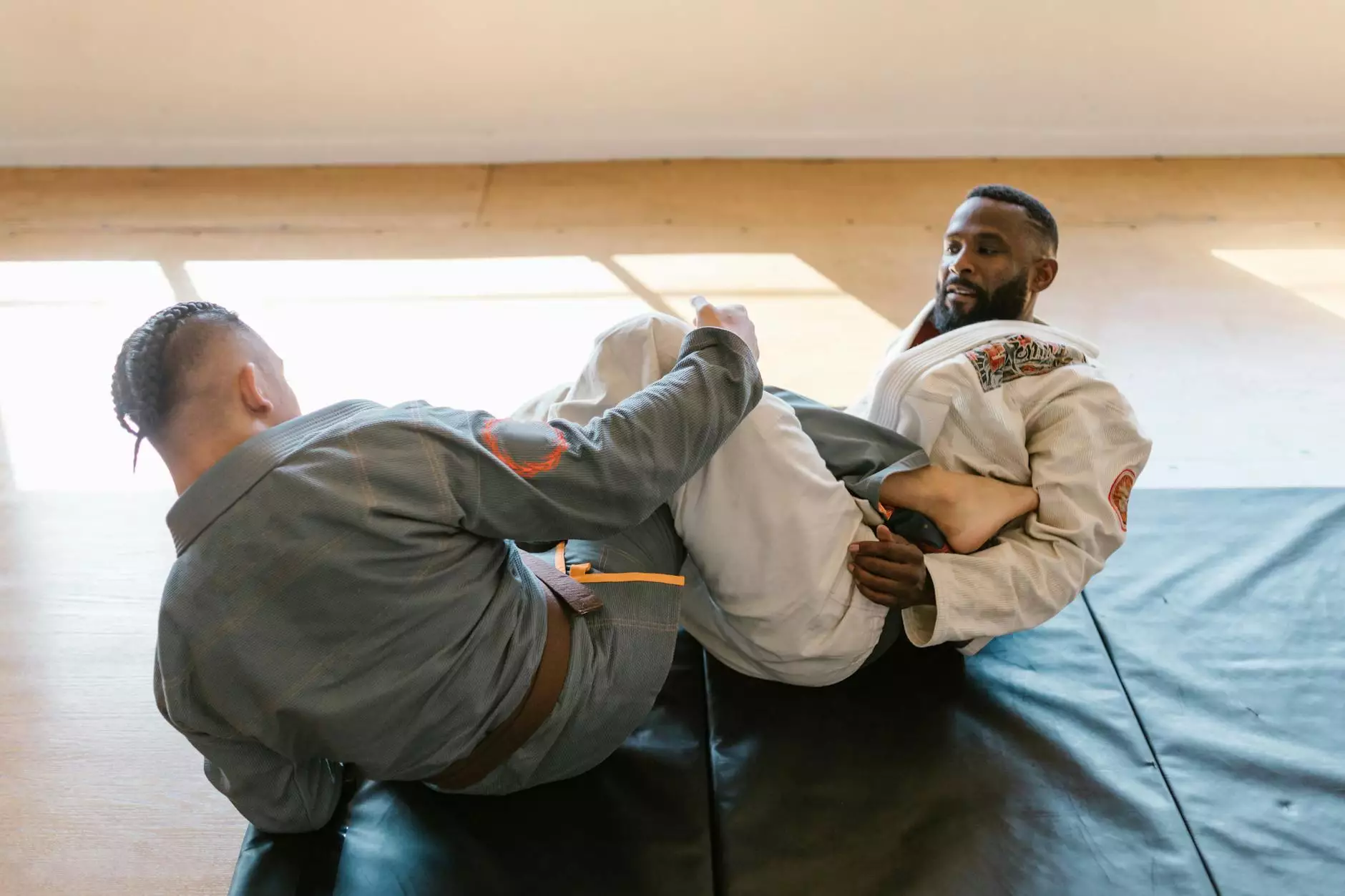
As technology continues to advance at an unprecedented pace, the development of self-driving cars has become a significant breakthrough in the automotive industry. With the potential to revolutionize transportation, self-driving cars rely heavily on a critical component known as training data. In this article, we will explore the importance of training data and its impact on the future of autonomous vehicles.
Understanding Training Data
Training data encompasses the vast amount of information that is needed to teach self-driving cars how to navigate and make intelligent decisions on the road. It consists of millions of data points collected from sensors, cameras, and other sources attached to the vehicle. These data points provide the necessary input for machine learning algorithms, enabling the car's artificial intelligence (AI) system to understand the environment it operates in.
Training data consists of various types, including images, videos, lidar point clouds, radar data, and more. Each type of data plays a crucial role in training the AI system to detect and recognize objects, understand traffic patterns, and respond appropriately in different scenarios.
The Significance of High-Quality Training Data
High-quality training data is paramount for the successful development and deployment of self-driving cars. The accuracy and reliability of the training data directly impact the car's ability to make safe and informed decisions while on the road.
Collecting and processing high-quality training data ensures that self-driving cars can accurately identify and interpret road signs, traffic lights, pedestrians, cyclists, and other vehicles. It also enables the AI system to predict and react to potential hazards and make informed decisions, ensuring the overall safety of passengers and other road users.
Furthermore, high-quality training data helps improve the performance of self-driving cars in various weather conditions, lighting situations, and complex driving scenarios. By exposing the AI system to an extensive range of real-world data, self-driving cars can adapt and learn from different situations, enhancing their overall reliability and performance.
Challenges in Training Data Collection
Collecting training data for self-driving cars poses several significant challenges. One of the main obstacles is the sheer volume of data required. The training data must cover various driving scenarios, including urban, suburban, and rural areas, all while accounting for different weather conditions and traffic patterns.
Another challenge lies in labeling the training data accurately. Each data point needs to be labeled with corresponding metadata, such as the type of object, its position, and relevant attributes. This process requires meticulous attention to detail, as even minor labeling errors can significantly impact the performance of the AI system.
Ensuring the diversity and representativeness of the training data is also crucial. Self-driving cars must be trained on a wide range of data, including different types of roads, intersections, and driving behaviors. By exposing the AI system to diverse training data, it can learn and adapt to a multitude of real-world scenarios, minimizing the risk of encountering unexpected situations on the road.
The Future of Training Data for Self-Driving Cars
As self-driving technology continues to progress, the demand for high-quality training data will only increase. The emergence of advanced sensors, such as high-resolution cameras and new lidar technologies, will provide even richer and more detailed training data, enabling self-driving cars to further improve their performance.
Innovations in data collection and processing techniques, including data augmentation and synthetic data generation, will also play a vital role in enhancing training data quality. These techniques enable the creation of additional training data by generating virtual scenarios and scenarios that are difficult to capture in real-world settings. This means that self-driving cars can be trained on a more extensive range of data, further refining their capabilities and reducing their reliance on real-world data collection.
Conclusion
Training data for self-driving cars is a crucial ingredient in enabling autonomous vehicles to navigate the roads safely and efficiently. High-quality training data, collected through various sensors and accurately labeled, forms the foundation for developing reliable AI systems that can interpret and respond to a wide range of real-world driving scenarios.
As the automotive industry continues to embrace self-driving technology, businesses like Keymakr.com, specializing in home services, keys, and locksmiths, play an essential role in supporting the growth and development of self-driving cars. Whether it's securing autonomous vehicles or providing solutions for key management, Keymakr.com ensures that the infrastructure surrounding self-driving cars is secure and efficient.
training data for self driving cars